Causal Inference and Machine Learning: The Current Frontier
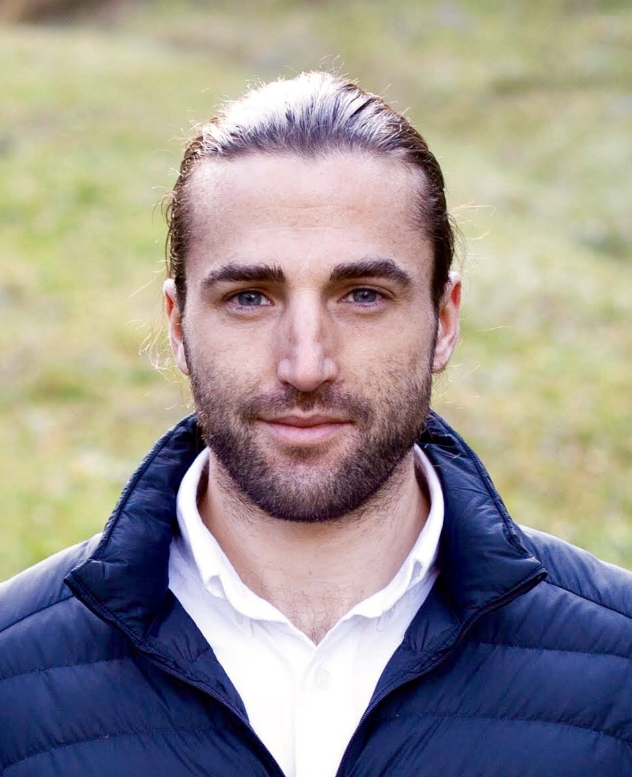
Gerard Torrats-Espinosa

Details
Machine learning is taking over the world, but it has an important but overlooked weakness: its output does not indicate causality. And causality, it turns out, is what lots of companies need to use analytical modeling for.
Lots of companies make large investments into their AI & ML teams without making this distinction, and it costs them dearly. Many of the largest tech companies have made large investments into their data science divisions with the precise goal of improving the amount of causal inference they can do on their business processes.
Causal inference is a powerful data science framework to make sense of the complex phenomena that we observe in the world around us. This talk will provide an overview of the fundamental concepts and applications of causal inference methods in domains where observational data are often used to assess causal relationships.
In the first part of the talk, we will explore traditional and contemporary methods of causal inference, including propensity score matching, instrumental variables, and regression discontinuity designs.
The second half of the talk will illustrate how machine learning methods, which often emphasize prediction, can be combined with the causal inference tools to tackle research questions in several domains. The talk will assume that attendees have a basic understanding of statistical inference and linear regression analysis.
While this event is FREE, tickets are required & space is limited!
Attend this event